The Halo Effect and Horn Effect are two common cognitive biases that significantly impact decision-making and perceptions in organizational behavior. Both of these biases affect how people evaluate others in the workplace, particularly in areas like performance appraisals, interviews, and daily interactions. Here’s an overview of each:
- Halo Effect
The Halo Effect occurs when an individual’s overall impression of a person influences specific judgments about their traits or behaviors. Essentially, if someone is viewed positively in one area, that positive impression can spill over into other areas, even unrelated ones.
- Example in Organizational Behavior: If a manager perceives an employee as hardworking and reliable due to a strong work ethic, they might also assume the employee is good at tasks like leadership or communication, even if there’s no evidence supporting that assumption.
- Implications: This bias can lead to overly positive performance evaluations, promotions based on perceived ability rather than actual performance, and favoritism in the workplace. It can also make managers overlook weaknesses in favored employees.
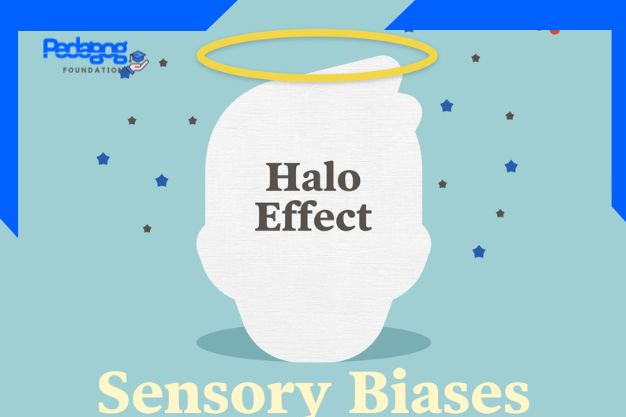
- Horn Effect
The Horn Effect is the opposite of the Halo Effect. It occurs when a negative impression about one trait of a person influences the perception of their other traits or behaviors.
- Example in Organizational Behavior: If an employee is late for work a few times, their manager might conclude that they are irresponsible or lazy in general, which may not be true. This negative perception can overshadow the employee’s positive qualities or contributions.
- Implications: The Horn Effect can lead to unfair performance reviews, a lack of development opportunities, and even conflict or poor team dynamics. Employees subject to this bias may feel demoralized or underappreciated, which can impact their performance.
Impact on Organizational Behavior:
- Performance Appraisals: Biases like these can skew evaluations. An employee’s entire review might be influenced by one particularly good or bad trait or incident, leading to inaccurate assessments of their overall performance.
- Hiring and Promotion Decisions: Managers might hire or promote someone based on a “halo” of good qualities rather than a comprehensive evaluation of their skills. Conversely, a “horn” might prevent a qualified person from being hired or promoted.
- Workplace Relationships: These biases can influence interpersonal relationships, leading to favoritism or unfair treatment within teams, causing resentment, poor collaboration, and low morale.
Organizations can mitigate these biases by implementing structured evaluations, fostering self-awareness, and training employees and managers to make more objective decisions.
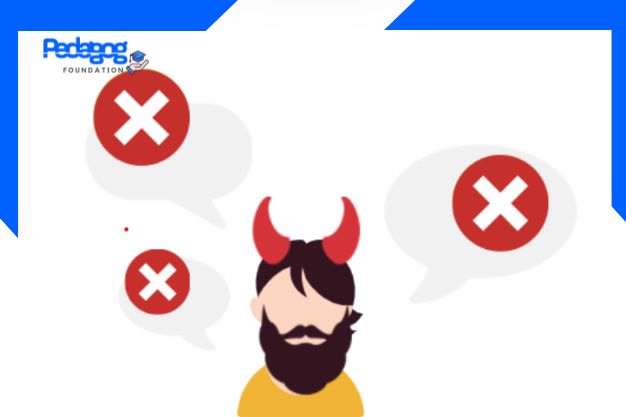
AI has the potential to help mitigate the Halo Effect and Horn Effect in organizational behavior, but it can’t eliminate these cognitive biases on its own. Here’s how AI could address the problem, along with some challenges and limitations:
How AI Can Help Solve the Halo and Horn Effects:
- Objective Data-Driven Decision-Making: AI can use objective, data-driven methods to evaluate employee performance, reducing the impact of subjective human biases. By analyzing a wide range of performance metrics, AI can provide a more balanced assessment of an individual’s contributions.
Example: In performance reviews, AI can track an employee’s productivity, work quality, and collaboration metrics, ensuring that the evaluation isn’t solely influenced by one positive or negative trait.
- Standardized Evaluation Systems: AI-powered systems can enforce consistent and standardized evaluation criteria for all employees. This reduces the chances of one employee being unfairly rated better (due to the Halo Effect) or worse (due to the Horn Effect) because every aspect of their performance is measured systematically.
Example: AI can structure interview or performance review questions so that everyone is evaluated based on the same set of competencies. - Continuous Monitoring and Feedback: AI can provide ongoing feedback based on real-time performance data, ensuring that evaluations are not based on isolated incidents or one-time impressions. This ensures a more holistic view of an employee’s capabilities.
Example: Instead of relying on a single performance appraisal at the end of the year, AI can track achievements, skill improvements, and feedback throughout the year. - Unbiased Screening in Hiring: AI can help in the hiring process by analyzing candidates’ skills, experiences, and qualifications without being influenced by personal impressions or subjective biases.
Example: AI can scan resumes and recommend candidates based on specific qualifications, not based on how they “appear” or initial first impressions. - Training and Development Recommendations: AI can identify specific areas of improvement based on employee data and offer personalized training or development suggestions. This ensures that weaknesses aren’t overlooked (Halo Effect) or overemphasized (Horn Effect).
Example: If an employee shows room for improvement in communication but excels in technical skills, AI can recommend targeted communication courses while recognizing their strengths.
Challenges and Limitations:
- Bias in AI Algorithms: While AI can reduce human bias, it is not immune to bias itself. If AI systems are trained on biased data or if the algorithm is flawed, the bias will be embedded in the system. For example, if past evaluations were influenced by Halo or Horn effects, AI could reinforce those biases.
- Over-Reliance on Quantitative Metrics: AI often relies heavily on quantifiable data, but certain qualities, such as creativity, leadership, or emotional intelligence, may be hard to measure accurately. This could lead to overly simplistic evaluations that miss important nuances.
- Lack of Human Insight: AI can make decisions based on data, but it may not fully grasp context, workplace dynamics, or personal challenges that affect an employee’s performance. For example, an employee who had a tough month due to personal reasons might be judged more harshly by AI.
- Ethical Considerations and Transparency: Employees may feel uncomfortable or distrust AI systems if they are not transparent about how decisions are made. If people do not understand how the AI evaluates them, it could create feelings of unfairness, even if the system is intended to be more objective.
Conclusion:
AI can certainly help reduce the effects of the Halo and Horn effects by using objective data, standardized criteria, and continuous feedback. However, it’s not a perfect solution and should be used in conjunction with human oversight to ensure fairness, context, and ethical decision-making. AI can be an effective tool to mitigate these biases, but organizations should ensure that AI systems are designed, trained, and monitored carefully to avoid introducing new forms of bias.
Submitted By
Dr. Rohit Kumar Pal
Senior Academician
Pedagog